While there's still much work to be done when it comes to the digitalization of construction, industry leaders are already developing and using artificial intelligence (AI) solutions with real business value. Among other things, AI improves occupational safety, increases predictability, and supports project management on construction sites with sensor and camera data.
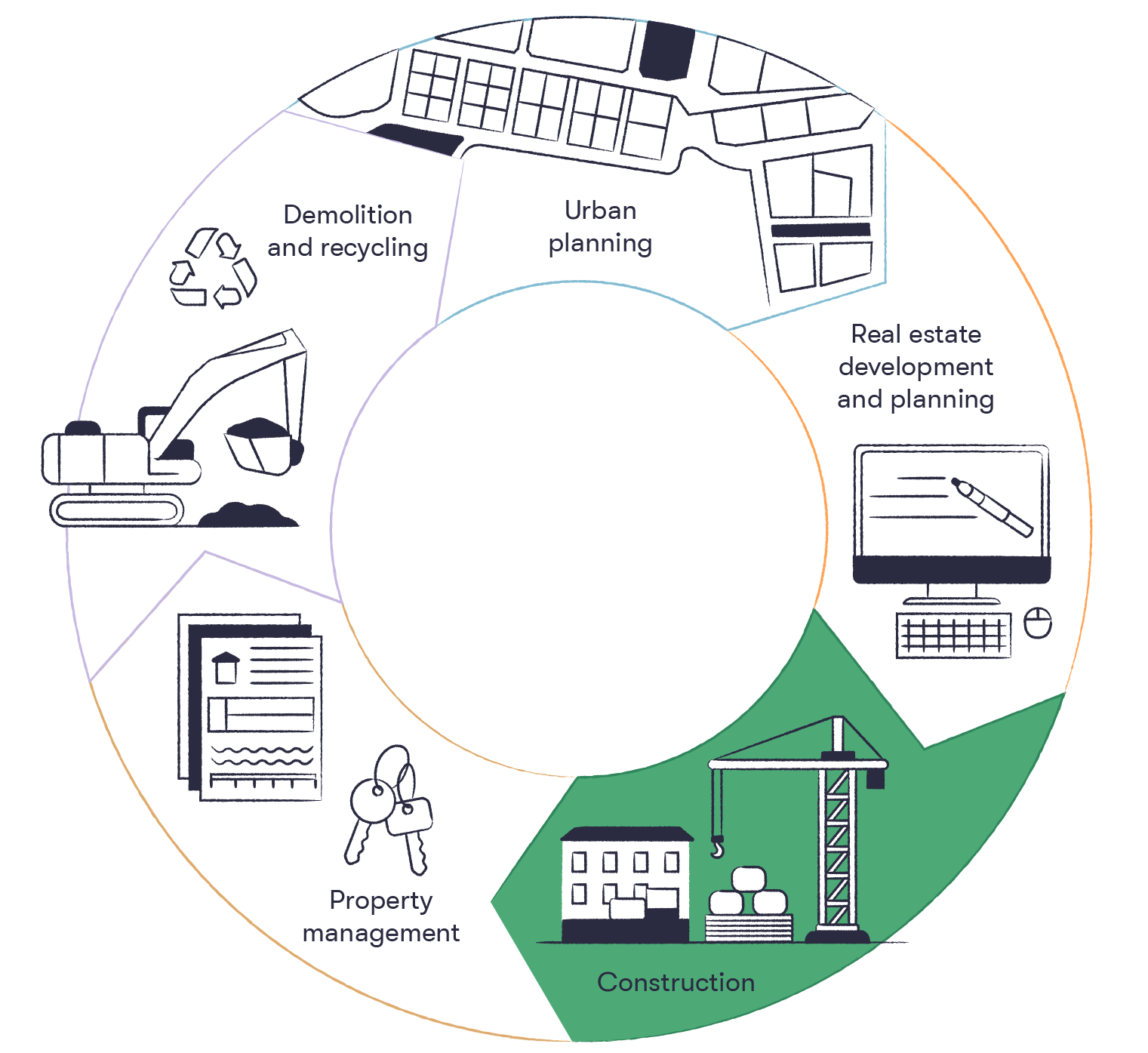
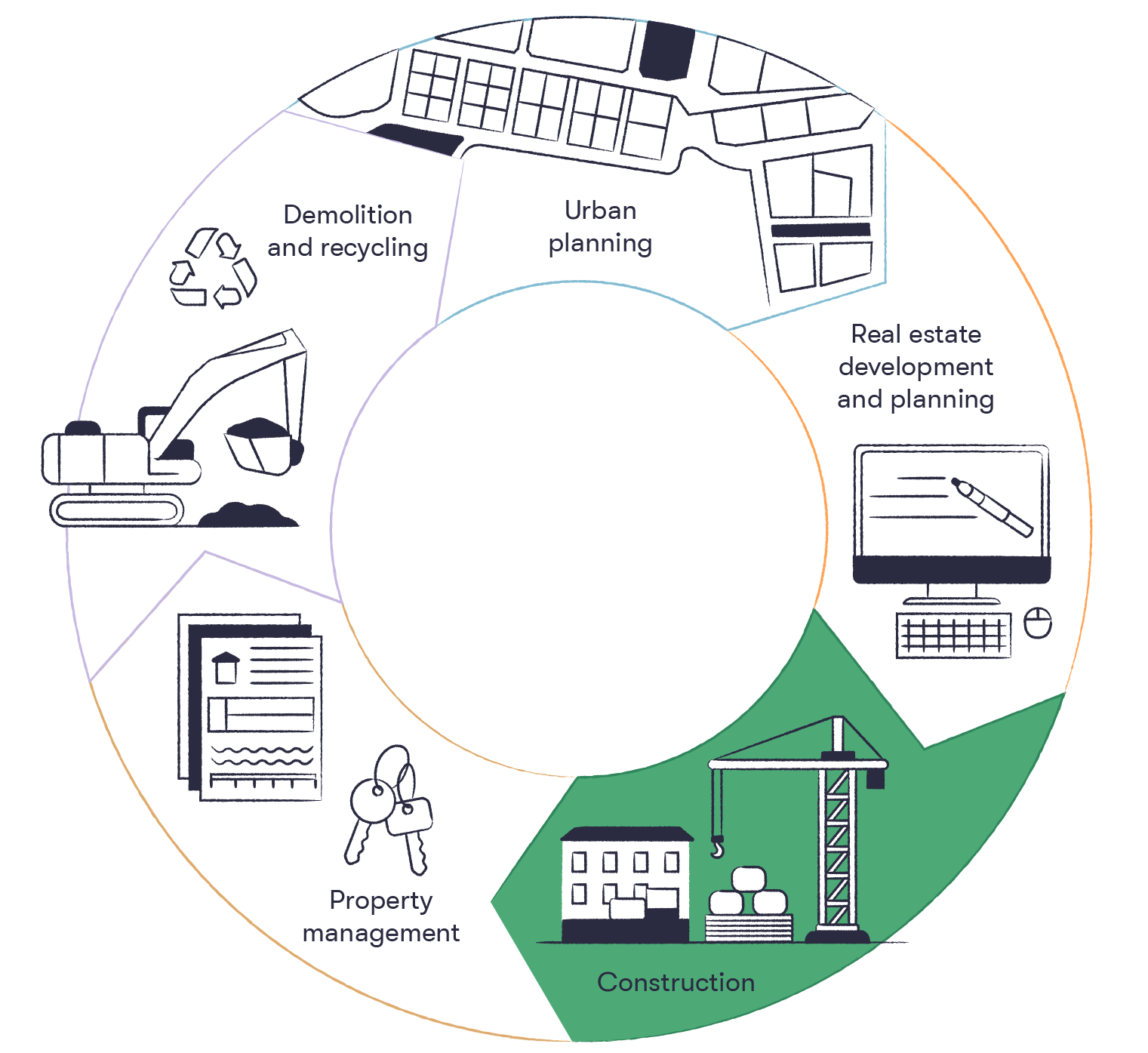
YIT – AI for sales and construction
YIT is a northern European urban developer and construction company employing around 7,400 professionals in ten countries.
AI development at YIT
Over the years, YIT has built several AI solutions. While many have remained in the test phase, a number have also entered production.
AI solutions are used in sales and site operations, especially for forecasting and clustering. Sales and site operations differ in their organization and ways of working.
Sales has common processes and good availability of data. Construction sites, on the other hand, are characterized by project specificity and varying availability of data. If different construction sites have different ways of working, it’s challenging to duplicate solutions from one site to another. That’s why YIT has applied AI in areas common to every construction site, such as occupational safety.
As a starting point, an AI solution always needs a process that can be automated, streamlined, or changed. In the absence of such processes, using AI is very difficult.
What makes an AI project successful?
The following is a list of YIT's prerequisites for success:
AI solutions must always support a company's strategically important business goals.
Businesses need to be committed and enthusiastic about promoting AI solutions. Otherwise, getting a solution into use can be challenging, even if the solution itself is good.
From the very beginning, it’s necessary to understand how the results will be distributed to end users (in other words, how the solution actually works in production).
Processes should be put in place to enable standardized data collection. The data collection process is the cornerstone of analytics and AI solutions.
When the data is seen by the parties producing it, for example through reporting or an application, they should validate it at the same time. Good visibility of data thus improves its quality. This also creates better starting material for AI development.
Not everything should be done in-house. Especially in support functions that exist across multiple companies (for example, finance, customer service, procurement, HR), it’s worth researching ready-made solutions and not immediately starting to develop your own.
Sign up to solve exercises
The quantity and quality of data is crucial
The most common reason for rejecting an AI idea at YIT has been either a lack or poor quality of data. In construction, data doesn't necessarily emerge by itself as a part of processes. Instead, data collection must be specifically planned and implemented. Often, data collection requires a new device like a camera or a sensor.
Construction sites are also constantly changing – literally from a pit to a building. The construction site's different phases, including their exterior and interior work, require the relocation of the cameras if data is to be collected during all phases of construction.
In other activities, such as sales, the quality and quantity of the data also affect the results, sometimes in a surprising way. For example, if a sales representative doesn’t always have time to fully record optional information about a deal in the sales system, a machine learning model for forecasting sales could conclude that withholding optional information most often leads to landing a deal.
Starting work on AI solutions too quickly without proper prior knowledge of data availability and quality is the most common reason for the solutions being delayed or rejected.
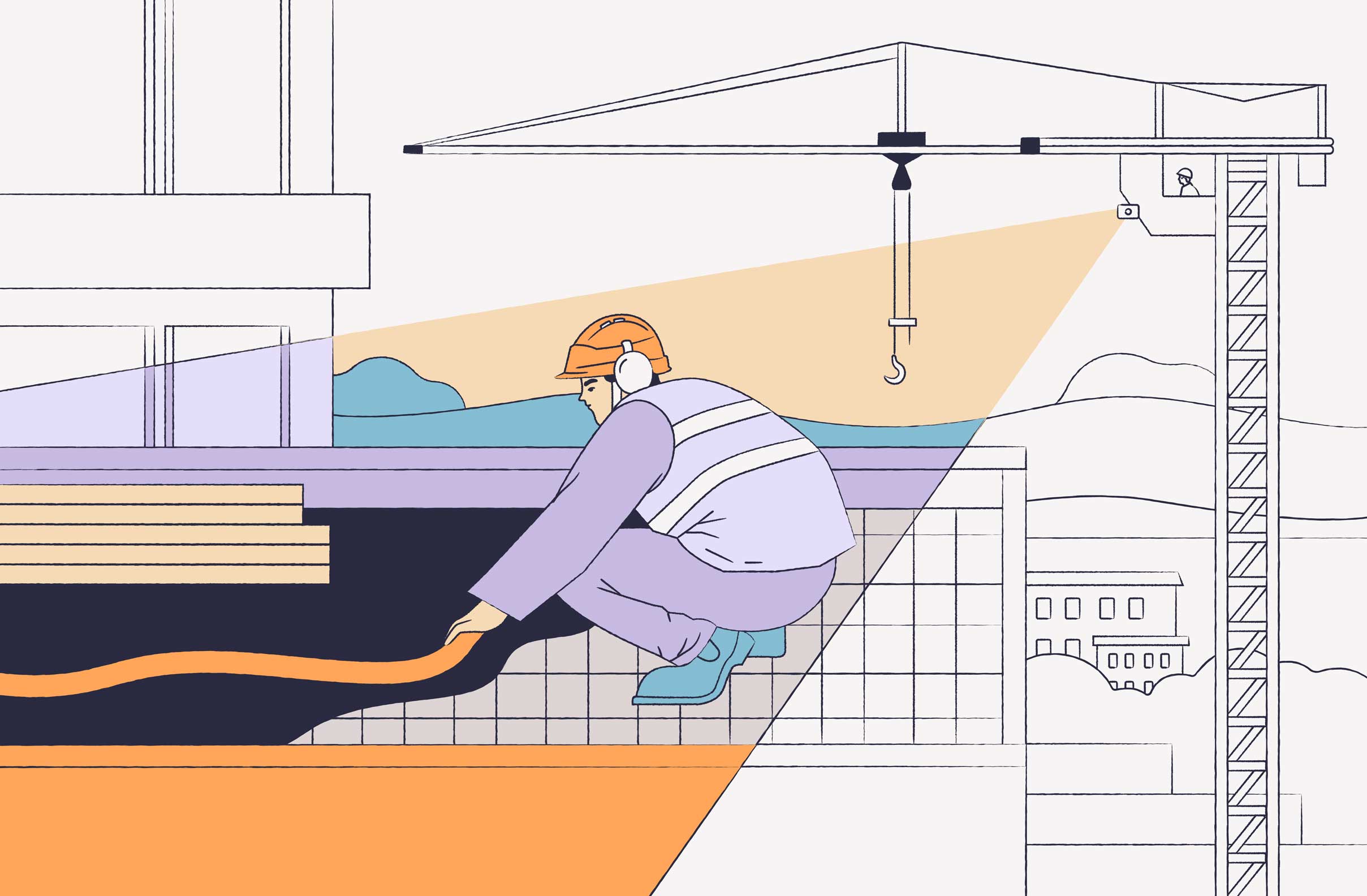
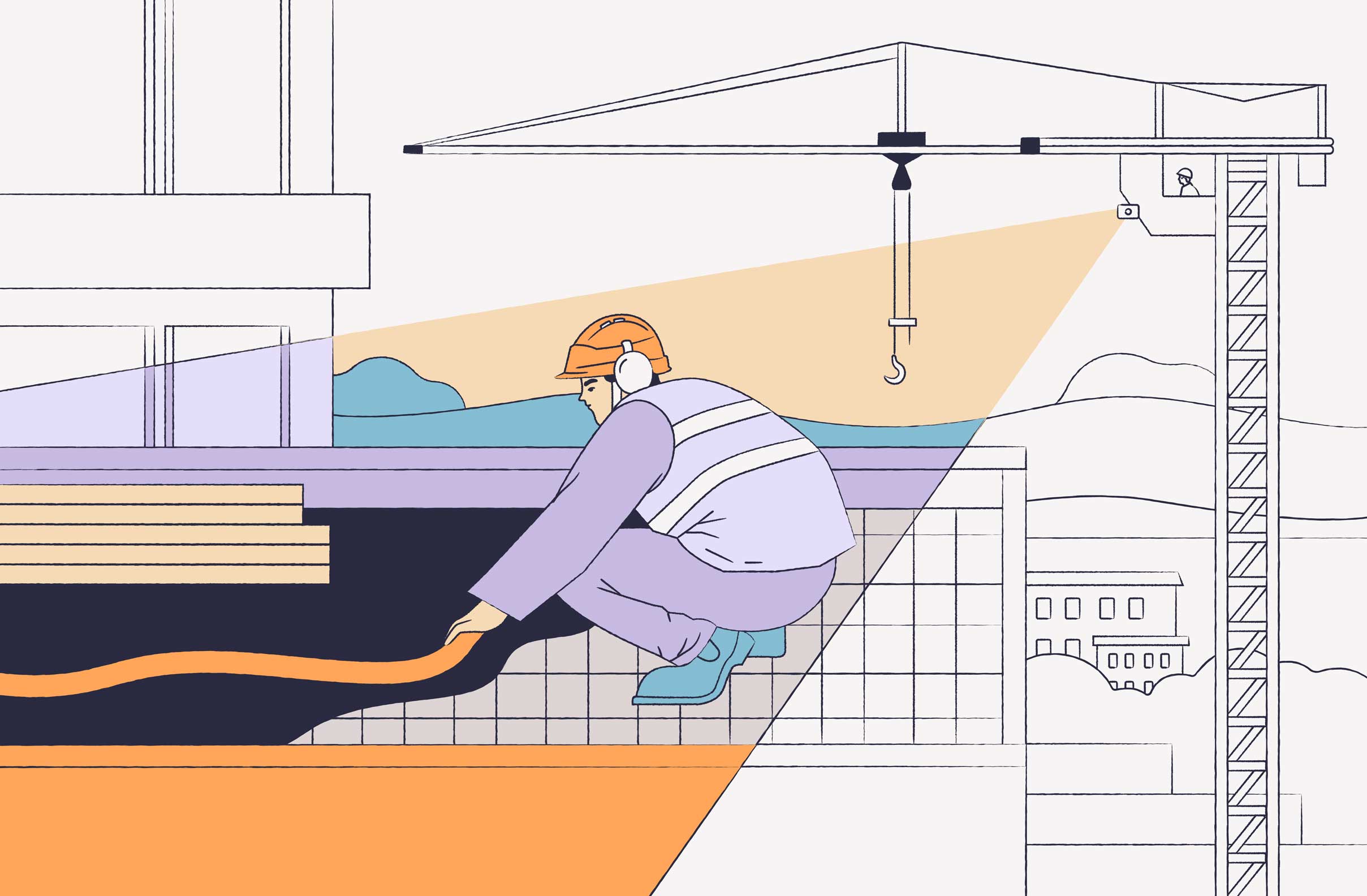
Identifying a construction site' work phase from camera images
Various machine vision solutions are used on construction sites. Together with Aalto University, YIT has tested how cameras can be used to identify the various stages of construction and ensure the construction site stays on schedule.
In the pilot project, a camera was installed on a construction site crane to capture the site. The machine learning model recognizes a phase such as “floor casting” or “reinforcement”. The solution provides a common picture of the site and thus speeds up the day-to-day monitoring of it, especially in large projects.
A common snapshot is just as important when building AI solutions. It’s not recommended to start working on the solution too quickly without answering the questions “why,” “how,” and “what.”
The following figure illustrates the areas YIT considered at the beginning of the AI project described above. The aim was to ensure the conditions for project success were considered comprehensively enough. This can be seen as one version of an AI canvas, which we'll explore in more detail in Chapter 3.
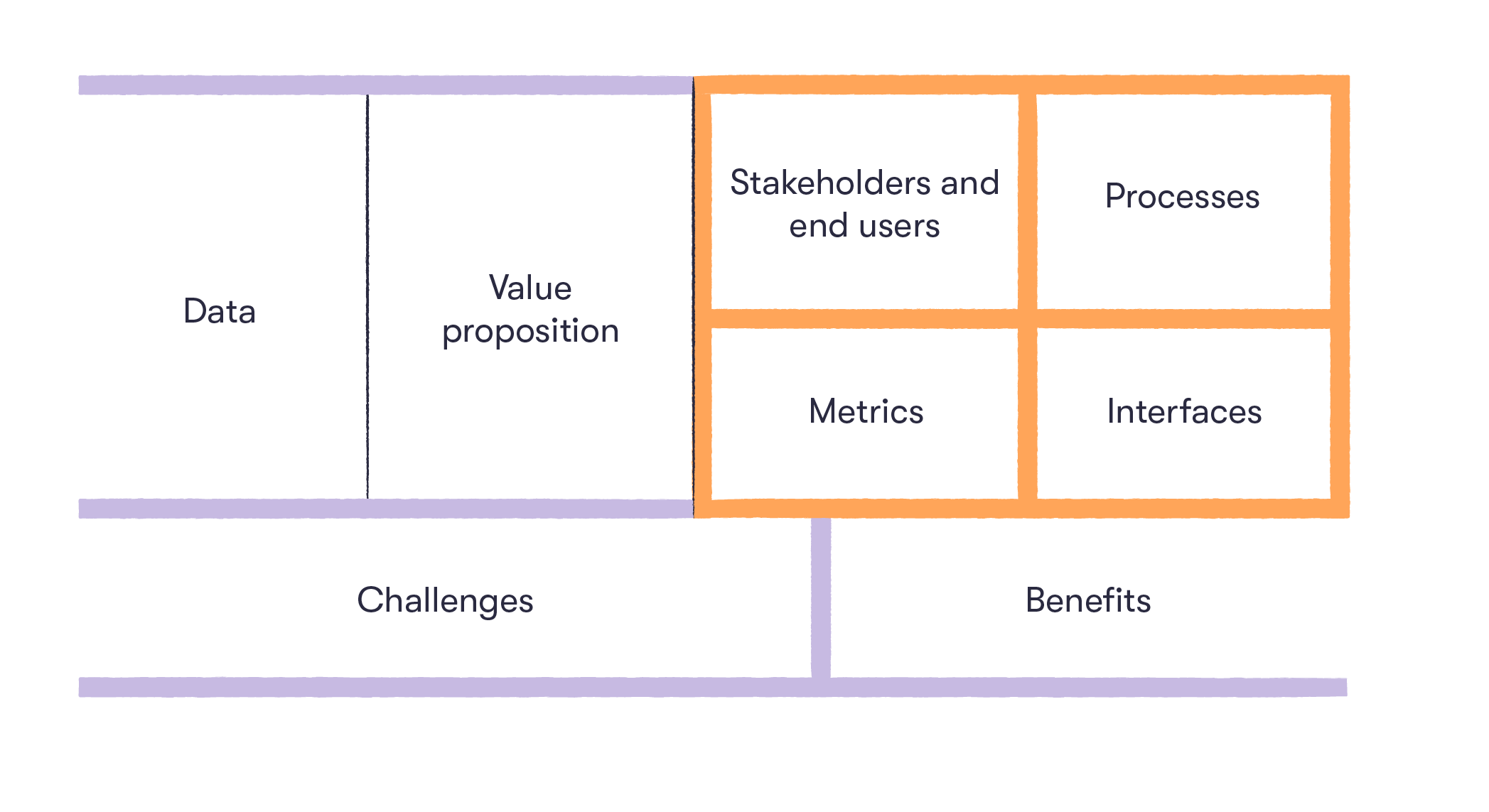
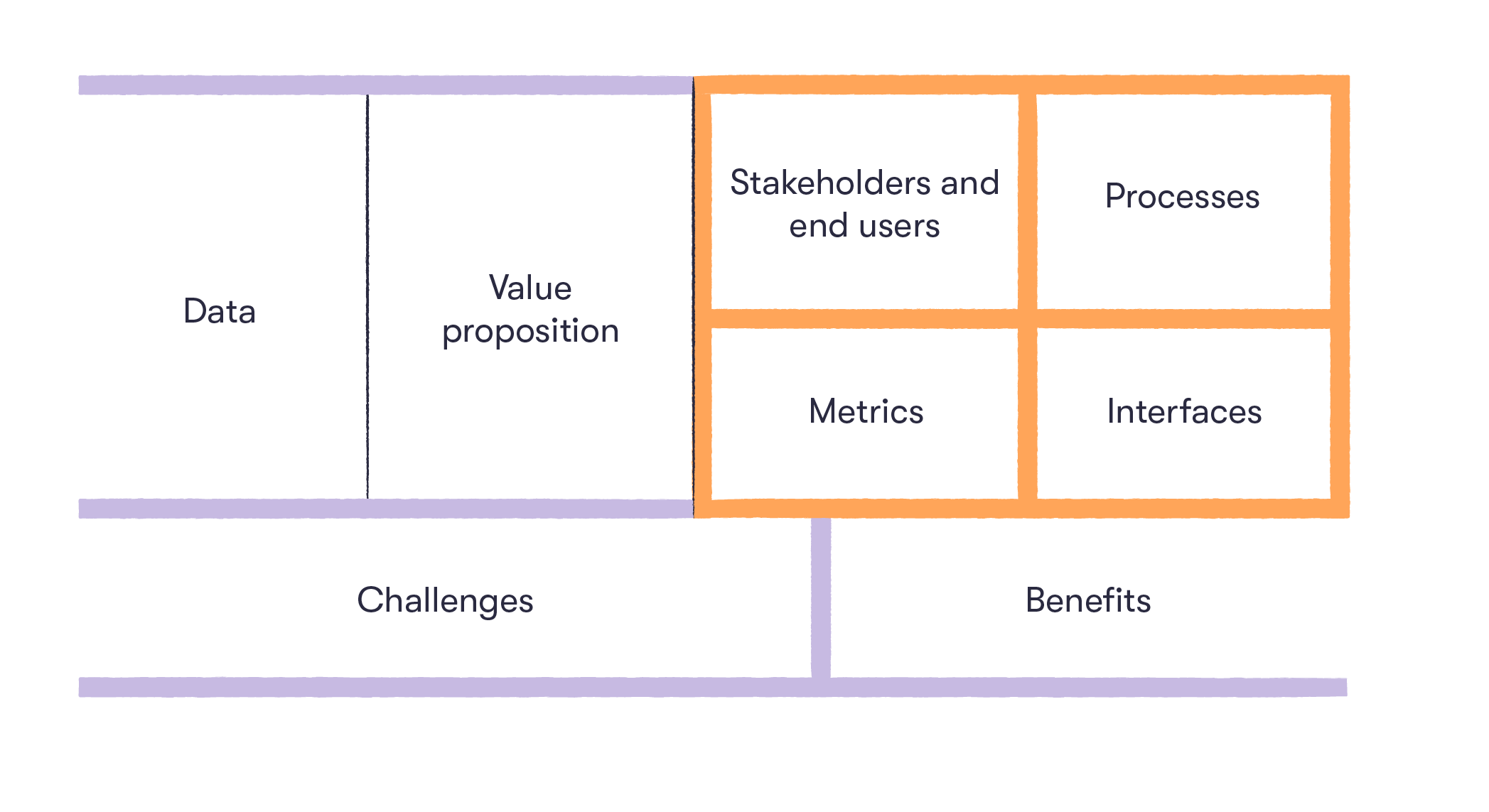
Sign up to solve exercises
A-Insinöörit – improving construction site communication
Large construction sites affect the lives of nearby residents, workers, and other people, often for several years. Still, a neighborhood has little influence over site plans, schedules, or traffic arrangements. Resident information, various surveys, and information do not reach all target groups equally.
Builders may be unaware of direct feedback from the environment and the external overall picture because channels and methods are decentralized. This often leads to problems being dealt with on social media or, more traditionally, in newspaper opinion pieces.
Internal communication on construction sites is also often deficient – information doesn't always flow quickly.
A-Insinöörit, working as the client's representative, decided to start solving the construction communication problem in the "Kalasatama to Pasila" tram alliance project. The role of communication is emphasized in a project that takes several years, is large in size, involves multiple stakeholders, and takes place in the middle of the country’s capital. With the experiment, A-Insinöörit hopes not only to increase the transparency of communication but also to understand the discussions better.
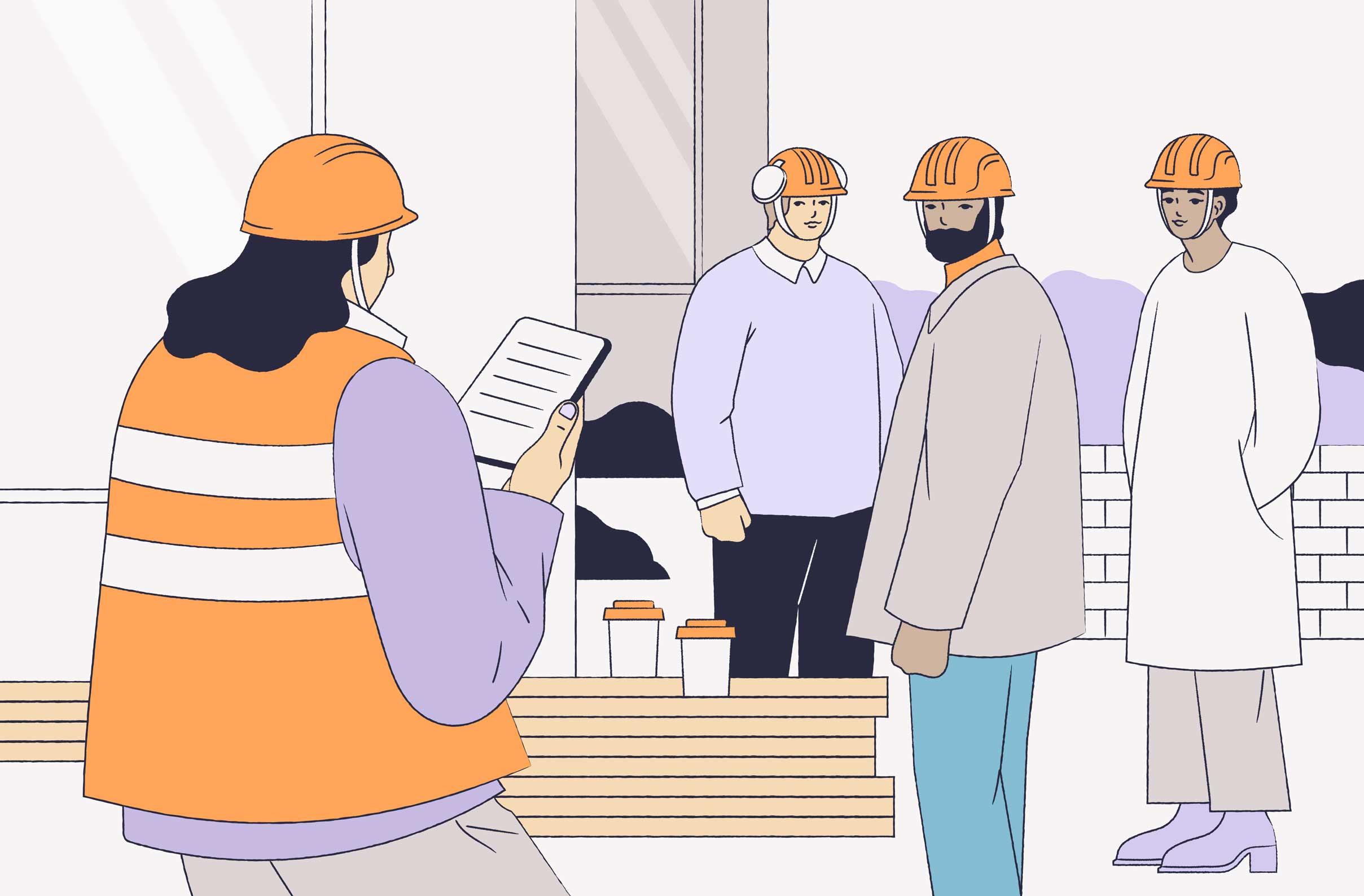
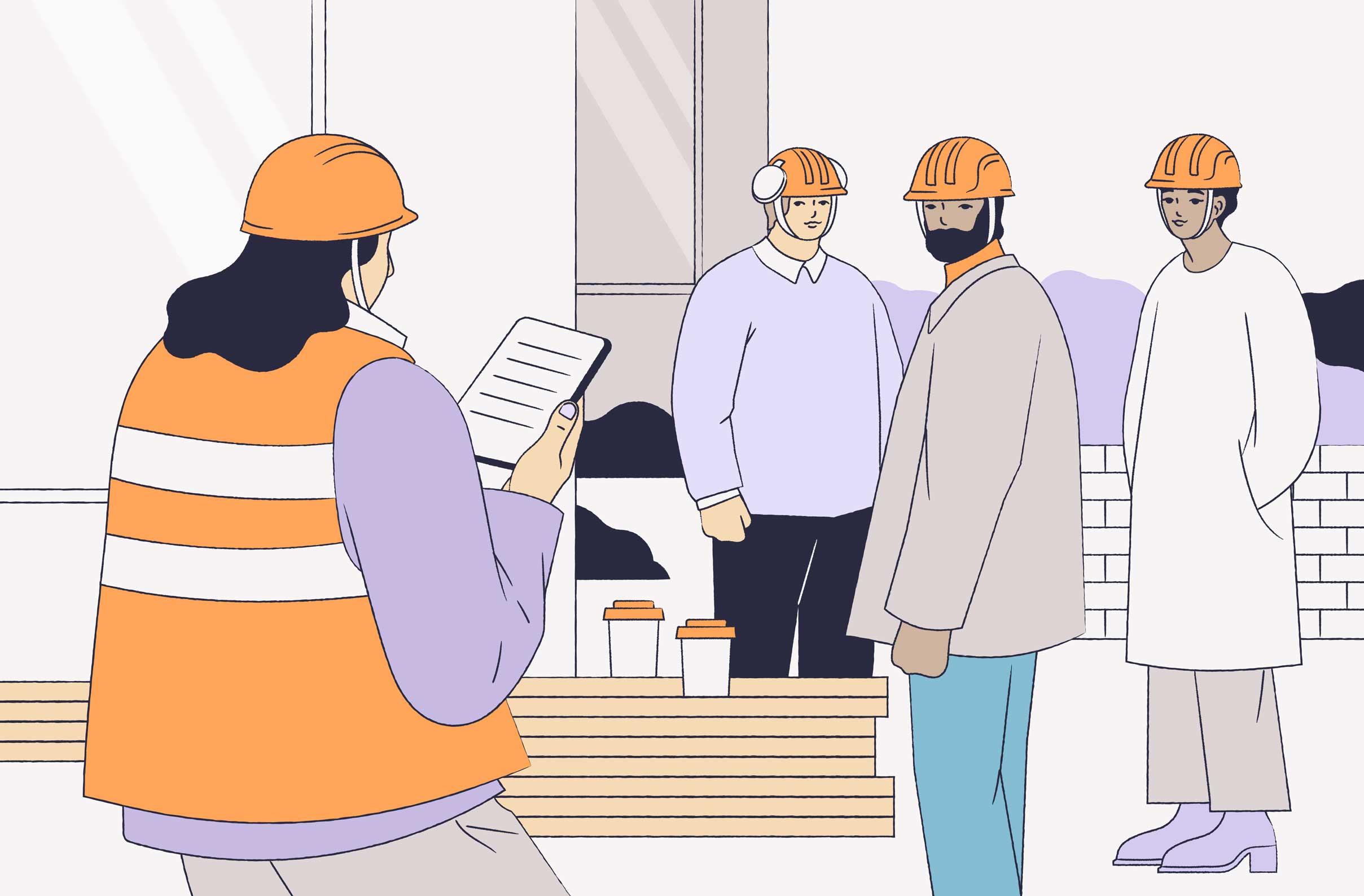
Mood analysis with AI
A-Insinöörit uses natural language processing (NLP) in the project's internal and external communication. The aim is to understand the trends, moods, and tone of conversations in written text.
Individual data sources include both external queries and customer feedback, and internal queries and feedback. Continuous data sources include discussions on the intranet and media monitoring. The data collected from these sources creates a visual snapshot that's constantly updated. The text analysis is performed by a Finnish service provider and the visual analysis is based on reporting software.
The solution gives the project's communication a more comprehensive picture of people's concerns. Accordingly, the project management receives information about the project team's mood, rising pain points, and job satisfaction. Based on this information, targeted internal and external communication can be conducted with the right groups on topics of interest to them.
An AI project promoting qualitative values
Contrary to the usual practice in AI projects, an immediate financial return can't be calculated for A-Insinöörit’s communication project. Instead, the return on investment is based on qualitative values such as reputation, work fluency, customer satisfaction, and the positive mood generated by listening to residents.
The project taught A-Insinöörit’s employees what AI is and how AI technologies can be used without large financial investments. The experiment was the company's first AI application in which external data sources were used directly in the solution.
The introduction of AI in companies should be thought of as a continuous learning process rather than a discrete investment. When weighing up the profitability of AI projects, the learning and development of understanding and their effects on the company's competitiveness must also be taken into account. Indeed, return on learning (ROL) is in many ways a better – or at least a complementary – measure alongside the traditional return on investment (ROI).